Fixxx
Moder
- Joined
- 20.08.24
- Messages
- 602
- Reaction score
- 1,830
- Points
- 93
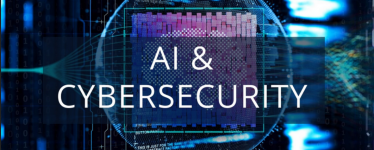
Neural networks are increasingly penetrating various aspects of our lives: from big data analysis, speech synthesis and image creation to managing autonomous vehicles and drones. In 2024 Tesla's creators added neural network support for autopilot and drone shows have long been using artificial intelligence to coordinate devices into various formations and display QR codes in the sky. Marketers and designers also apply AI in their work to generate illustrations and text. Following the release of ChatGPT in late 2022 and its popularity various companies started actively developing their services based on GPT models. With the help of different AI-based services and Telegram bots neural networks have become accessible to a wide range of users. However, if information security rules are not followed the use of various services and neural networks poses certain risks.
Threats and Risks of Using Neural Networks for Users.
The euphoria sparked by the introduction of GPT chat for many people has turned into caution. With the emergence of numerous services based on language models, both free and paid, users have noticed that chatbots can provide inaccurate or harmful information. Particularly dangerous is false information related to health, nutrition, finances as well as instructions on weapon manufacturing, drug distribution and more. Furthermore, the capabilities of neural networks are constantly expanding and the latest versions can create remarkably realistic fakes, synthesizing voices or videos. Scammers exploit these functions to deceive their victims by forging messages and calls from their acquaintances and creating videos with famous personalities. For example, in 2021 a video created by a neural network allegedly featuring Oleg Tinkov (a recognized foreign agent in Russia) promoting investments spread on social media. Upon clicking the link next to the video a fake bank website opens. Over time it becomes increasingly challenging to verify counterfeit content. To protect users from this threat the Ministry of Digital Development, Communications and Mass Media plans to create a platform to detect deepfakes.
The main threat is the emerging trust of many users in neural networks and chatbots in particular. Neural networks are often perceived as accurate and unbiased but people forget that AI can operate on fictional facts, provide inaccurate information and draw erroneous conclusions. It has been shown multiple times that mistakes happen. If you ask idle questions, the damage is likely minimal. However, if you use chatbots to address financial or medical matters the consequences could be severe. Moreover, often to receive a response from a neural network you need to provide certain data. The big question is how this data will be processed and stored afterward. There's no guarantee that the information you include in your queries will not resurface in the dark web or become grounds for a quality phishing attack.
Training a neural network can be done with a trainer, autonomously or in a mixed manner. Self-training has its strengths but also drawbacks - malicious actors find ways to deceive neural networks. Within LLM two main types of vulnerabilities that can affect system users: vulnerabilities related to the model itself and vulnerabilities in the application where this model is used. Some models can continuously learn based on information from their users. This process allows the model to be continuously refined. However, there's a downside to this process. In cases of model configuration errors, it's potentially possible for user A to request the model to return questions that user B previously asked. As a result, the model might provide this information since it has essentially become part of the shared database operated by LLM.
Attackers may exploit vulnerabilities in neural networks to obtain user's confidential data. They use various methods to attack chatbots and language models. For example, they employ "Prompt Injection" a method that involves altering existing prompts or adding new ones. As a result of this attack the neural network may process data incorrectly and produce erroneous results. Currently, the most notable vulnerability is "prompt injection" where specific requests to a neural network formulated in a particular way grant access to the network's internal data which the developer may be unaware of. Quite recently a chatbot from Microsoft disclosed highly sensitive information after just one query. In March 2024 bug hunters from the Offensive AI Lab discovered a way to decrypt and read intercepted responses due to the data encryption feature in ChatGPT and Microsoft Copilot. The method wasn't precise enough and OpenAI responded to the report and fixed the issue. Attackers also exploit vulnerabilities in APIs to steal confidential data including passwords or corporate information. Additionally, vulnerabilities enable DDoS attacks on the system and bypassing security measures.
The second global area of vulnerabilities is associated with the application that utilizes the neural network. Large Language Models (LLMs) cannot function independently and require some external "wrapper" to enable users to interact with them. Typically, this "wrapper" is a web application or service that provides end-users with a convenient mechanism for formulating queries. These applications may be susceptible to all classic vulnerabilities that potential attackers can exploit to steal personal data and user's chat histories.
Another example of an attack on chatbots is SQL injection. In this scenario attackers can gain access to the service's databases or inject malicious code into the chatbot server. There are several types of attacks on AI and it's essential to distinguish between them. For instance, attacks can involve evasion, poisoning, trojans, reprogramming and extracting hidden information. Speaking of the significance of breaches, evasion attacks (modifying input data) are potentially the most common. If a model requires input data to function, attackers may attempt to modify the data in a way that disrupts the AI. On the other hand data poisoning attacks have a long-lasting impact. A trojan present in an AI model persists even after retraining. All these can be categorized as adversarial attacks - a way to deceive a neural network into producing incorrect results. Neural networks are still not adequately protected from attacks, data tampering and interference in their operations for malicious purposes. Therefore, users should exercise caution and follow specific guidelines when working with chatbots.
Precautionary Measures and Recommendations.
The technology of large language models is rapidly advancing deeply integrating into everyday life and attracting more users.
To protect yourself and your data from potential threats when interacting with neural networks it's crucial to adhere to certain rules:
- Don't share confidential information with chatbots.
- Double-check the information provided by the chatbot.
- Download neural network applications from trusted sources.
Conclusion.
Both businesses and individual users have already experienced the benefits of neural networks. They help address everyday tasks, save time and money in various areas. For example, generative neural networks have significantly impacted the cost of producing movies, series and other videos that require graphics and processing. However, the same neural networks have also led to a wave of deepfakes such as the new variation of the Fake Boss attack. Every user should understand that neural networks are vulnerable. Like a messenger, email account or task scheduler - they can be hacked or susceptible to other malfunctions. Therefore, it's essential to approach working with them thoughtfully.
Last edited: